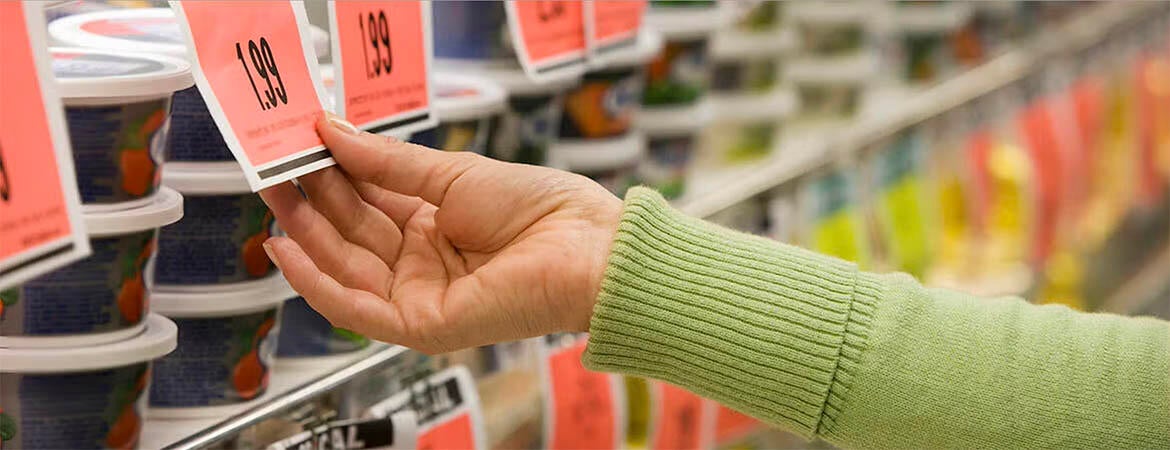
Setting the right price for goods or services is essential for just about any business. Prices that are too low can reduce profits. Prices that are too high can drive away customers and lead to losses.
Artificial intelligence (AI) deep learning models can help businesses find the elusive sweet spot by extrapolating prices from historical sales data, which generally show that sales go down as prices go up. But these predictions become unreliable when circumstances differ from the time the source data was generated, such as when the COVID-19 pandemic disrupted manufacturing supply chains and drastically altered consumer demands.
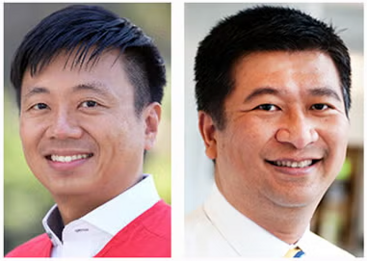
In a recent study, UC Riverside School of Business professors Mingyu “Max” Joo and Hai Che and their collaborators from Baruch College and Ohio State University have solved this problem by developing a deep learning model that considers both historical sales data and the economic theory of demand. Economic theory of demand accounts for factors such as income levels, consumer preferences, and consumption patterns under various circumstances such as holidays or extreme events like pandemics and natural disasters.
Such a combination allows AI to quantify the unpredictable aspects of how prices affect consumer behavior during unprecedented circumstances, explained Joo, an associate professor of marketing.
“With the help of economic theory, we could better identify demand fluctuations driven by external factors, like a pandemic or holiday fever, versus pure price responses,” Joo said. “This differentiation is crucial for making more reliable predictions.”
For example, traveler demands for hotel rooms are higher during the peak summer months despite higher rates. A standard AI model may mis-predict that higher prices are associated only with higher demands. However, the higher demands are driven by better weather or work schedule constraints, and consumers’ affordability limitations and sense of price fairness will still limit how much they are willing to pay. Such factors are difficult to gauge during uncertain times, Joo said.
The new model bridges a gap between standard AI models and the real-world complexities that businesses face when setting prices, particularly in unprecedented scenarios such as economic shocks and extreme price fluctuations.
To validate their model, the researchers analyzed pre- and post-COVID-19 retail data for breakfast cereals, which had a sales surge during the beginning of the pandemic, but later returned to a pattern of historic sales declines. They compared their new model combining economic theory with standard deep learning and log-linear models and evaluated their ability to predict demand changes as prices fluctuated beyond historical ranges.
The results were compelling. While standard models performed well with data within the known range, their predictions deviated when confronted with post-pandemic price levels. The researchers’ model, however, retained high accuracy, demonstrating a substantial improvement over other methods by reducing generalization errors by up to 50% in some cases. Such errors occur when a model trained on a specific dataset does not perfectly capture the underlying patterns or relationships that hold in different contexts.
“The pandemic was a perfect stress test for our model,” Joo said. “The price and demand patterns during COVID-19 differed significantly from any prior period. This was exactly the type of scenario where typical AI models would struggle to produce accurate forecasts.”
While most AI models that rely heavily on past price data falter when circumstances change, the new model’s ability to utilize economic theory gave it an edge, Joo said.
“We’re combining the best of both worlds—advanced AI techniques and established economic principles—to create a system that’s both intelligent and adaptable,” Joo said. “The future of AI in business isn’t just about exploiting more data; it’s also about leveraging human knowledge from various domains to build smarter, more reliable tools.”
The model is detailed in a paper titled “Theory-Regularized Deep Learning for Demand-Curve Estimation and Prediction.” In addition to Joo and Che, the authors are Chul Kim of the Zicklin School of Business at Baruch College, CUNY; and Dong Soo Kim of the Fisher College of Business at Ohio State University.
It was presented last year at the Proceedings of the IEEE International Conference on AI for Business in Laguna Hills.